One of the big lessons of cheap talk models is to exert some degree of skepticism when the incentives of those doing the talk, and often sharing mostly unverifiable opinions, are not fully aligned with ours. Recently, President Biden, Fed Reserve Jerome Powell and the NBER took the historically unprecedented step to refuse to call at two-quarter decline in GDP a “recession.” Reasons given are, well, bizarre, and reminding us well of the market for excuses that Watts and Zimmerman originally described.
“It’s just supply shocks,” as if decline in activity did not hurt the economy if these are due to exogenous causes. Under this definition, the first oil shocks of the 70s would not qualify as a recession, nor any of the post-war shocks after the economy returned to normalcy.
“it’s temporary,” as if the recessions had to be permanent to matter and there was a well-accepted definition of how temporary a shock needs to be.
“it’s ok if unemployment is low;” so, to be clear, the theory is that a recession must demonstrate a labor market inefficiency, and, if, say, willingness to work unexpectedly decreased to the point of dramatically reducing output, this is NOT a recession.
The response is very much like a person cheating on their spouse and explaining it shouldn’t be called cheating in the first place because (1) it’s just a shock to the supply of attractive partner, (2) it’s temporary until the fling passes, and (3) it’s ok because that’s what they wanted to do in the first place.
To be sure, all current players have own specific agendas. Biden wants to keep confidence to complete his political promises, Powell is an ex-investment banker trying to keep to the covid stock market and real estate bubbles alive, and the NBER is paranoid about creating a self-fulfilling recession if there is any remote chance it might be avoided. Perhaps, among the three, the NBER response is the most disappointing, as its leadership is no longer acting as a pure disinterested research institute telling us about average events based on prior established science but making new calls about the societal consequences of its findings and the word it uses, without the rigorous process of academic documentation.
An important disclaimer: I am not a macroeconomist, and my perspective has been greatly influenced by taking the neo-classical macro courses taught by Finn Kydland and Ben McCallum at Carnegie Mellon as a grad student. They are among the most scholarly individuals I have ever met, and think about the science as its own goal, not as means for persuasion. Macro is one of the most complex subsets of economics that I have seen, crossing micro, aggregation, complex systems, and all of this with limited correlated data that require solid models to fill the gaps. So, my objective here is not to make any sort of deep analysis. However, what these scholars also teach is that macro is also a matter of common-sense approach to concrete facts and behaviors, jointly informed by data and theory. To this effect, I will share three plots of fundamental economic quantities taught by these economists that conflict with the current spin of policy makers. Let me also point to the excellent blog by NYU’s Nouriel Rubini’s blog, who, unlike me, is a legit macroeconomist, and has been pointing to many of these worrisome aspects.
The first plot below is the monetary aggregates M1 and M2, and the CPI inflation (from the St Louis Fed). For reference, M1 is mainly money plus checking and savings accounts, while M2 includes money markets (short-term debt) that are almost equivalent but not immediately tradable. The CPI inflation is for all consumer goods in the US cities, including energy.
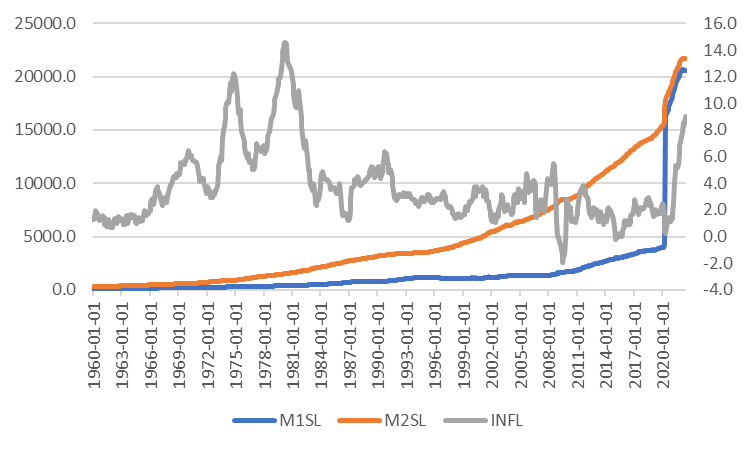
A link between M1 and M2 is expressed by the Friedman money equation which states, below, that:
M x V = P x Y
and is interpreted as money velocity (M) times volume of money (V) being equal to price (P) times real output (Y).
At its core, the equation is an accounting tautology given that velocity V is simply the amount of transactions P Y divided by the amount of money (for example, V is one is all money circulates once). However, behind this simple principle, it helps organize the Friedman monetarist doctrine. We shouldn’t have any reason to expect long term changes to propensities to keep idle cash balances (V should stay constant), and it makes sense to have a rate of inflation low to avoid productive disruptions (P should stay approximately constant or grow slowly). Hence, M should grow at the rate of output Y, say, about 3% a year.
A unique aspect of the Covid pandemic is that V abruptly decreased because most consumers could not engage in normal purchases, implying abnormally low (temporary) decrease in inflation. At the same time, this was offset by an enormous increase in money supply (see plot above), which avoided deflation. Post Covid, money velocity will be returning to its pre-Covid levels so, from Friedman’s equation, we can simply infer the rate of cumulative implied inflation as the amount of growth in M1 and M2. Relative to January 2020, M1 grew by a whopping 414% and M2 grew by 41%. Using the more aggressive estimate of M2, we shouldn’t be expecting just 10% inflation over a few months, but sustained inflation over multiple years.
Unless of course, the Fed removes from circulation the liquidity. But note that this is not done via interest policy but by open market repurchases, which the Fed has repeatedly delayed and, to be honest with ourselves, would create a major economic breakdown as there would be no one else to buy short-term debt. Repurchasing such large amounts of debt is just not a feasible policy in any near future unless we see measurable productivity gains.
By way of an anecdote, I was giving a talk in a Central Bank earlier this year, and asked the economists why they were still predicting low levels of inflation despite the fact that money supply had exploded to levels that would justify a cumulative abnormal rise in inflation between 30% and 40% (or even more under M1). They pointed out that they did not believe in the Friedman model, because the Great recession of 2008 showed enormous increases in the money supply with no effect on inflation. In fact, they also admitted that central banks no longer used economic models with money being explicitly modelled (as per the models taught by McCallum) but instead had a real economy with inflation coming up from an exogenous relationship with unemployment and output gap. This model is useful to understand the real economy, but is it an appropriate model when the main concern is predicting inflation? It isn’t, nor it is being used for that purpose. The current inflation predictions we see being circulated are primarily driven by statistics, not economic theory. Statistics do not work for unique events for which past data may not be relevant and one has to understand the core economic equations of a problem.

The second plot is about the asset bubble that is currently under way. During covid, asset markets exploded despite widely documented decrease in productivity (which we all have experienced) during the stay-at-home mandate. It is possible that a year or two of productivity may have been lost, and possibly more if many workers have gotten used to a less effective type of work. Wall Street never corrects for inflation so all earnings per share earnings growth numbers include inflation. Many firms are reporting spectacular near two-digit growth in earnings.
Let us note that the model presented here is right: in my financial statements analysis course, I ask students to use nominal cost of capital rates to discount future cash flows, without any correction for inflation, so the cash flows should be apples to apples without any adjustment for inflation. So, the high earnings growth is good news, no? Besides, aren’t real assets a cover for inflation? But that’s not true empirically, as asset markets have done worse during periods of high inflation (especially throughout the seventies). The problem is that real assets indeed do very well during periods of unexpected short-term inflation because the cost of capital does not immediately adjust to higher inflation. However, if inflation is long-term, as the plot suggests, the risk-free rate will increase by approximately the expected additional inflation to cover the shortfall.
The plot above is a calculation value using the Gordon growth formula. The assumption is that there is an asset paying $1 in the first year growing at 3% per year, and whose value is calculated by discounting at a risk premium of 5% plus the risk-free rate. The risk-free rate is then varied from zero to 20%. Conservatively, the long-term interest rate was about 3% until the recent period (much lower in the EU) and may be about 4% higher if the 30-40% cumulative inflation is spread out over a long horizon. In principle, the net effect of an increase in interest rate exactly matching the additional inflation is zero, so asset markets should not respond to increase in earnings due to inflation (although the market capitalization should increase by the rate of inflation). Except that markets have consistently responded positively to earnings news driven solely by inflation!
So a more direct calculation is to evaluate the effect of the market realizing that the increase in earnings will be matched by an offsetting increase in interest rates – something that is not fully reflected in prices at the moment. Comparing 3% to 7% implies a 40% readjustment in the value of assets – more or less the difference between peak post Covid markets and pre-Covid levels. The market is thus quite far from pricing the consequences of inflation on interest rates.
The last plot is perhaps the most important. It has been repeatedly stated that unemployment is low, which in turn is incompatible with a recession. Both neo-classical and neo-Keynesian models have reasons for changes in unemployment. In the former, these can be caused by shocks to total factor productivity and, in the latter, there are many possible shocks including shocks to relatively disutility of labor. If unemployment is low, then this should mean that employment is high, no? Well, not exactly, as the plot below shows.
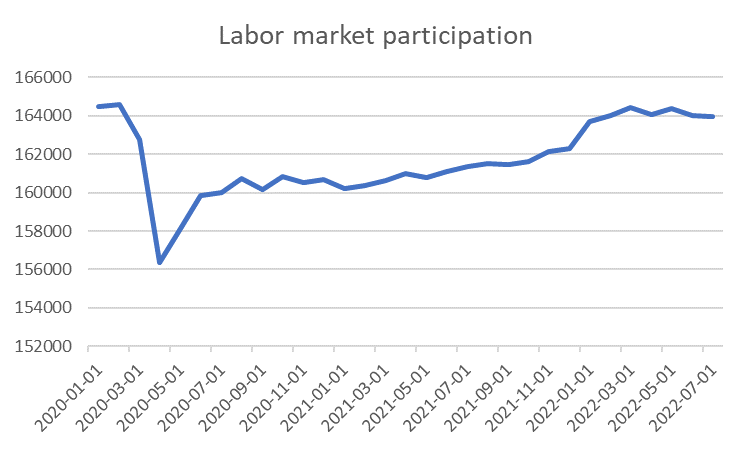
Labor market participation is lower than what it was in January 2020. The low unemployment rate is due to phenomena we have known as the Great Resignation or changes in working habits. To compare apples to apples, consider the following calculation. From 2010 to 2020, labor market participation grew by 7.3%, which implies that it should have been (1+0.073)^(1.5/10) higher than Jan 2020 under normal conditions in July 2022, this amounts to 166.3 million, versus 164 million currently, so about 2.3 million people are not being counted as unemployed because they no longer officially declare themselves as unemployed (whether they are looking for jobs is a different question). The unemployment rate is currently 3.5%, but if we include this labor force, by adding 2.3 to the current total of 5.9 million unemployed, we obtain a corrected unemployment rate of (2.3+5.9)/166227=4.9%, which is larger than historical averages and well within accepted ranges for the start of a recession.